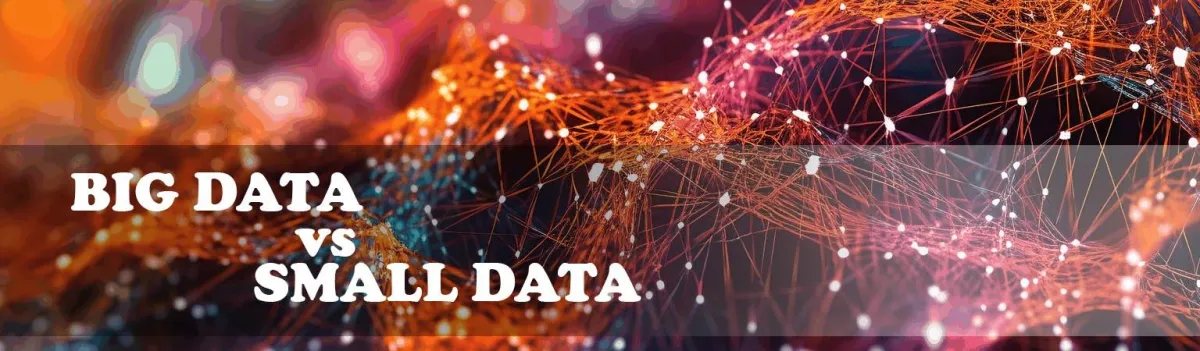
Big Data vs Small Data: Choosing the Right Approach for Your Business in 2024
In today's data-driven business landscape, companies are inundated with information from various sources. The challenge lies not just in collecting data, but in effectively analyzing and leveraging it to drive business growth. This is where the concepts of big data and small data come into play. As a leading IT solutions provider, Nexus System understands the importance of choosing the right data approach for your business. Let's dive into the world of big data and small data to help you make an informed decision.
Understanding Big Data and Small Data
What is Big Data?
Big data refers to extremely large and complex datasets that cannot be effectively processed using traditional data management tools. Characteristics of big data include:
Volume: Massive amounts of data
Velocity: High speed of data generation and processing
Variety: Diverse types of structured and unstructured data
Veracity: Uncertainty and reliability of data
Value: The potential insights and benefits derived from the data
What is Small Data?
Small data, on the other hand, focuses on smaller, more manageable datasets that are easily understandable and actionable. Key features of small data include:
Specific and targeted information
Easily processed using traditional tools
Often collected over shorter time periods
Typically focused on a particular aspect of business or customer behavior
The Power of Big Data
Advantages of Big Data
Comprehensive Insights: Big data allows businesses to analyze vast amounts of information from multiple sources, providing a holistic view of operations and customer behavior.
Predictive Analytics: With big data, companies can identify patterns and trends to make accurate predictions about future outcomes.
Enhanced Decision Making: Big data analytics can uncover hidden correlations, leading to more informed business decisions.
Improved Customer Experience: By analyzing large datasets, businesses can personalize offerings and improve customer satisfaction.
Challenges of Big Data
Resource Intensive: Processing and analyzing big data requires significant computational power and specialized skills.
Data Quality Issues: With large volumes of data, ensuring accuracy and reliability can be challenging.
Privacy Concerns: Collecting and analyzing vast amounts of personal data raises important privacy and security issues.
Complexity: Interpreting results from big data analysis can be complex and time-consuming.
The Value of Small Data
Advantages of Small Data
Accessibility: Small data is more manageable and accessible, especially for small to medium-sized businesses.
Quick Insights: Analysis of small data can provide rapid, actionable insights for immediate decision-making.
Cost-Effective: Small data analysis typically requires less investment in tools and expertise.
Focused Approach: Small data allows businesses to concentrate on specific, relevant information without getting lost in excess data.
Challenges of Small Data
Limited Scope: Small data may not provide a comprehensive view of complex business operations or customer behavior.
Potential for Bias: Smaller datasets can be more susceptible to sampling bias, potentially leading to inaccurate conclusions.
Scalability Issues: As businesses grow, small data approaches may become insufficient for handling increased data volumes.
Choosing the Right Approach for Your Business
Selecting between big data and small data depends on various factors:
Business Size and Complexity: Larger enterprises with complex operations may benefit more from big data, while smaller businesses might find small data more manageable.
Industry and Market: Some industries, like finance or healthcare, may require big data analytics due to regulatory requirements and the complexity of operations.
Available Resources: Consider your budget, technical capabilities, and human resources when choosing between big data and small data approaches.
Business Goals: Align your data strategy with your specific business objectives. Some goals may require comprehensive big data analysis, while others can be achieved with targeted small data insights.
Data Sources: Evaluate the types and volumes of data your business generates or has access to.
Bridging the Gap: Hybrid Approaches
Many successful businesses are adopting hybrid approaches that combine elements of both big data and small data:
Start Small, Scale Up: Begin with small data analysis and gradually incorporate big data techniques as your business grows.
Targeted Big Data: Use big data analytics for specific, high-impact areas of your business while relying on small data for day-to-day operations.
Small Data for Validation: Use small data approaches to validate and refine insights gained from big data analysis.
The Role of Artificial Intelligence and Machine Learning
Advancements in AI and machine learning are revolutionizing both big data and small data analytics:
Automated Data Processing: AI can help process and analyze large datasets more efficiently, making big data more accessible to smaller businesses.
Enhanced Small Data Analysis: Machine learning algorithms can extract more value from small datasets, improving the accuracy and relevance of insights.
Predictive Modeling: AI-powered predictive models can work with both big and small data to forecast trends and outcomes.
Making Data Work for You
In the end, the choice between big data and small data isn't always an either/or decision. The key is to understand your business needs, available resources, and long-term goals. Many businesses find success in starting with small data approaches and gradually incorporating big data techniques as they grow.